Can Large Language Models Retain the Magic of Harry Potter?
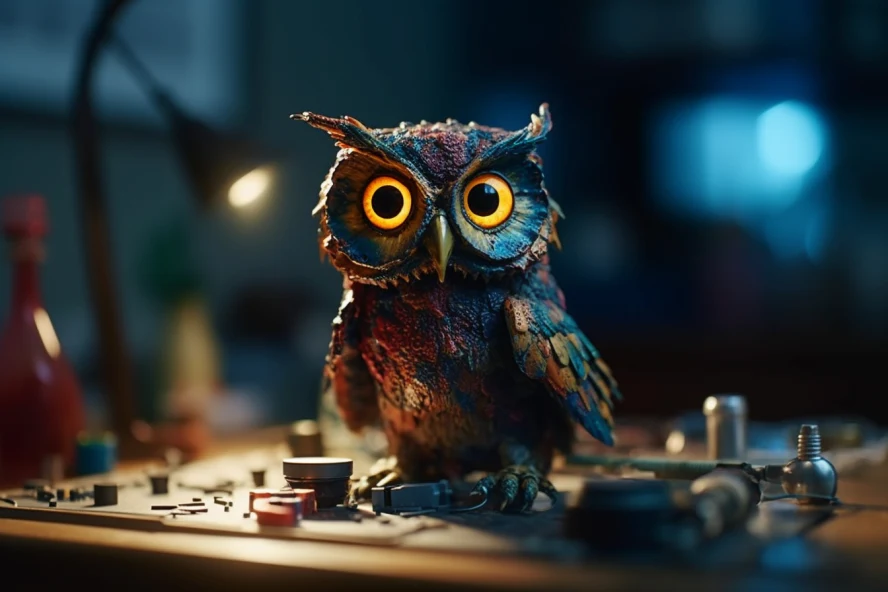
Large language models (LLMs) have swiftly ascended as pivotal drivers in the realm of artificial intelligence, catalysing breakthroughs and heightened efficiency across numerous sectors. These sophisticated models, sculpted by comprehensive training on extensive and varied datasets, are now indispensable tools for companies eager to exploit the full spectrum of AI's potential. At the core of leveraging these advanced systems lies a critical question: Is it possible to effectively modify or reverse their training without compromising their dependability and functionality? For instance, is it possible to reprogram a model to selectively forget specific data, such as the details of the Harry Potter series?
Reprogramming an LLM to forget Hogwarts
Addressing this challenge, the process of targeted knowledge elimination or modification within LLMs presents a unique opportunity to significantly enhance their versatility and criticality in AI-driven business applications. Cutting-edge research, venturing into uncharted techniques and methodologies, is showing promising avenues for resolving this intricate issue, potentially leading to the development of LLMs that are more custom-tailored and adaptable.
The Baked Cake Analogy
Just as flour is thoroughly mixed and integrated into a cake, information in LLMs is deeply embedded into their complex network of neurons. The training of these models involves adjusting millions or even billions of parameters based on vast datasets. This intricate nature of knowledge within LLMs makes the task of extracting specific information extremely challenging, as it would be to try to extract flour from a baked cake.
When attempting to remove flour from a cake, one faces the challenge of precision. Similarly, in unlearning, the goal is to selectively forget or remove certain information without disturbing the overall structure and functionality of the model. This requires precise techniques and algorithms that can target specific knowledge without degrading the model's performance on unrelated tasks. There's a delicate balance to maintain; removing too much information or doing it incorrectly could lead to a loss of general proficiency or the introduction of new biases.
After the unlearning process, it's crucial to evaluate the model to ensure that only the targeted information is lost. This is akin to checking if the cake still holds up without the flour, in terms of taste and structure.
Technical Methodology
To provide a technical overview of the methodology described in the paper "Who’s Harry Potter? Approximate Unlearning in LLMs," let's delve into the three main components of the technique, its implementation, and the underlying principles.
1 - Reinforced Model Creation and Logit Comparison
Firstly, the technique involves training a special version of the AI model, which we refer to as the 'reinforced model'. This is done by giving it extra training on specific content, such as the Harry Potter series. The model then learns to identify key words or phrases (which are actually called tokens in this context) linked to this content. The unique aspect here is comparing the model's responses before and after this extra training. This comparison helps us identify which tokens are most associated with the specific content, like filtering out key ingredients in a recipe to understand their significance.
2 - Generating Generic Predictions
The next step is about teaching the AI to replace specific terms from the target content with more general ones. This is achieved through two methods:
- Reinforcement Bootstrapping: This method involves retraining the AI on the specific content it initially learned – in our example, the Harry Potter series. However, this time, the focus isn't on learning everything anew but rather on identifying words that don't significantly change in meaning or importance, even in the context of Harry Potter. Suppose the model initially learned the word 'wizard' from the Harry Potter series. In reinforcement bootstrapping, it might be realised that the word 'wizard' can also apply broadly to other fantasy contexts and isn't unique to Harry Potter. Therefore, 'wizard' would be considered a 'generic' term and kept for use in general scenarios.
- Anchored Terms Method: Here, the AI is taught to swap out specific terms from the Harry Potter content with more general ones. This doesn't mean changing the meaning but rather replacing unique identifiers with broader terms. The AI might replace 'Hogwarts' with 'school' or 'Harry Potter' with 'the boy.' So, when it encounters a sentence like "Harry Potter went to Hogwarts," it's trained to generate a response as if it read, "The boy went to school." This way, the AI learns to respond with more generic terms, distancing itself from the specific Harry Potter content.
3 - Fine-Tuning on Alternative Labels
This phase involves adjusting the AI's training to focus on these newly identified generic labels or terms. The goal is to make the AI 'forget' the specific details from the original content and use the new, generic information instead.
Initially, if the AI model is asked, "Who is Dumbledore?" it might respond with specific details like, "Dumbledore is the headmaster of Hogwarts in the Harry Potter series, known for his wisdom and power."
After the fine-tuning process, where the AI is trained to forget specific Harry Potter-related information, its response might be a general statement like, "I'm not sure who that is." This shows that the AI no longer recognises 'Dumbledore' as a specific character from Harry Potter, indicating that it has effectively unlearned that specific piece of information.
Simplifying the Technical Process
In essence, this process involves identifying the specific information to be forgotten, training the AI to shift its focus towards the corresponding ‘generic version information, and then reinforcing this new ‘generic’ knowledge. It's a delicate balance between removing certain knowledge and maintaining the model's general understanding and capabilities. This approach highlights the technical complexity of AI learning processes and opens up new possibilities for managing AI knowledge in a controlled manner.
Business Implications
The concept of unlearning in AI, especially in the context of Large Language Models (LLMs), opens up numerous business applications that can transform how companies interact with AI technology. Here's an expanded view on how businesses can leverage unlearning:
- Ensuring Legal Compliance by Adapting to Changing Laws and Regulations: As laws and regulations evolve, especially concerning data privacy and intellectual property, businesses must ensure their AI systems comply with these changes. Unlearning provides a mechanism for AI systems to adapt to new legal frameworks without the need for complete retraining, thereby saving time and resources.
- Responding to Right-to-Be-Forgotten Requests: With regulations like the GDPR, individuals have the right to request the deletion of their personal data. Unlearning enables businesses to comply with such requests in their AI systems, ensuring that specific personal data is effectively removed from the model's knowledge base.
- Protecting Intellectual Property: If a business inadvertently trains its AI systems on copyrighted material, unlearning offers a way to remove this content, thus avoiding legal repercussions and respecting intellectual property rights.
- Securing Competitive Advantage: In industries where proprietary knowledge is a key asset, companies can use unlearning to ensure that their AI systems do not retain sensitive information that could give competitors an edge if leaked or inadvertently shared.
- Maintaining Ethical AI Practices: Unlearning can be used to remove biases that AI systems might have acquired during training. This is crucial for businesses aiming to demonstrate their commitment to ethical AI practices and to avoid public relations issues related to biassed AI decisions.
- Customising AI Content: In scenarios where businesses need to tailor their AI systems for specific cultural or societal contexts, unlearning can be used to remove irrelevant or potentially offensive content, ensuring that the AI's output aligns with the desired values and norms.
The implementation of unlearning in AI presents a versatile tool for businesses, allowing them to navigate the complex landscape of legal compliance, intellectual property management, ethical AI practices, and data privacy. As AI continues to integrate deeply into business operations, the ability to selectively unlearn information will become increasingly vital, offering a pathway to more responsible, adaptable, and legally compliant AI solutions.
Final Thoughts
As we explore whether large language models (LLMs) can 'forget' specifics like the Harry Potter series, we realise the importance of AI unlearning in the realm of ethical artificial intelligence. This emerging technology, still in its early stages, is pivotal for adhering to regulations such as the GDPR. It not only facilitates the effective handling of Right-to-Be-Forgotten requests, ensuring personal data removal, but also plays a crucial role in eliminating biases acquired during training. Each advancement in AI unlearning brings us closer to an era where AI is not only powerful but also ethical and adaptable, signifying a major step forward in the responsible evolution of AI technology.
Let us solve your impossible problem
Speak to one of our industry specialists about how Artificial Intelligence can help solve your impossible problem
